The Rise Of Generative AI And Its Effects On Video Platforms
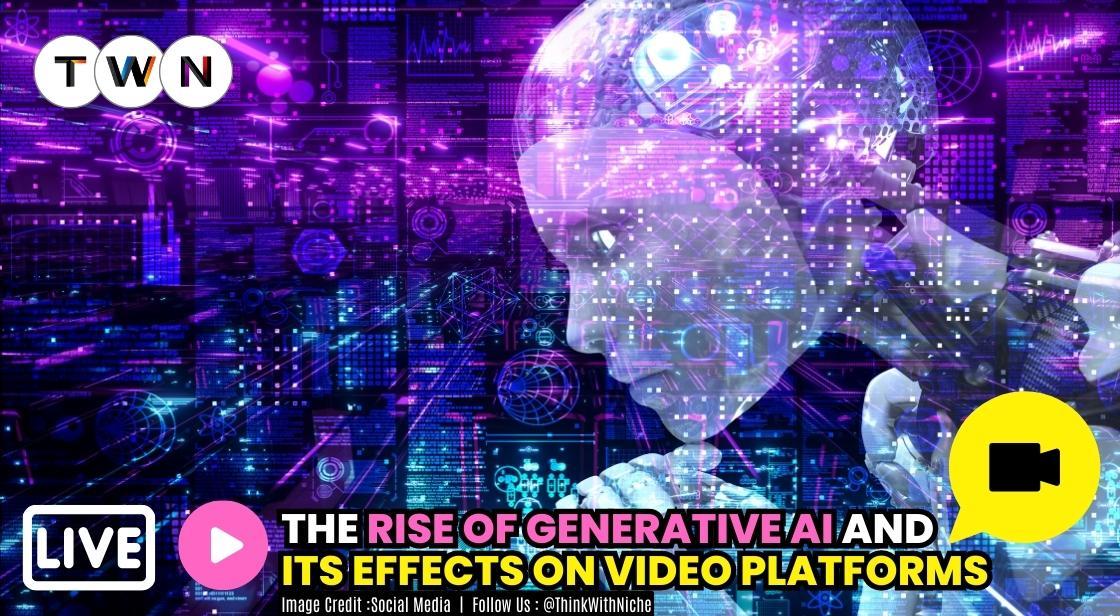
Blog Post
An artificial intelligence model called generative AI is able to produce text, images, audio, and video by predicting the next word or pixel after being trained on enormous datasets. A text description is the most basic input (sometimes referred to as a prompt) for generative AI. A text-to-image model like Stable Diffusion, a generative pre-trained transformer (GPT), MusicLM, and Imagen Video may all produce audio and visual content based on a text description. This technology will make all forms of content creation more accessible.
Overall, generative AI has the potential to revolutionize video platforms by enabling the creation of new and engaging content at scale. By learning from the vast amounts of data available on these platforms, generative AI can help to automate content creation, reduce production costs, and increase the diversity and quality of content available to viewers. However, as with any new technology, there are also potential risks and challenges that must be carefully considered and managed.
It might equalise the playing field for video production more than smartphones and social video platforms already have. The video content sector will likewise experience a profound transformation as a result. This article will provide a detailed analysis of the Rise of Generative AI and Its Effects on Video Platforms.
Generative AI is rapidly transforming the video industry by creating personalized content, automating content creation, enhancing search and discovery, improving advertising, and increasing engagement. As a result, video platforms are able to keep up with the increasing demand for content and stay ahead of the competition. However, the rise of generative AI also brings challenges, such as the potential for bias and the need for ethical considerations. It is crucial for the industry to navigate these challenges to fully realize the potential of generative AI in video platforms.
In recent years, the field of artificial intelligence has grown by leaps and bounds. One of the most exciting and innovative developments in this field is generative AI, which has the potential to revolutionize many industries, including video platforms. In this article, we will explore the rise of generative AI and its effects on video platforms.
What is Generative AI?
Generative AI is a type of machine learning that involves the creation of new content by a computer. Unlike other forms of AI, which are designed to recognize patterns and make predictions, generative AI can actually create new content from scratch.Generative AI relies on neural networks to generate new content that is similar in style and format to existing data. For example, a generative AI model trained on a dataset of images can create new images that resemble those in the original dataset. Similarly, a generative AI model trained on a dataset of text can generate new sentences or paragraphs that are similar in style and tone to the original text.
How Does Generative AI Work?
Generative AI works by analyzing large amounts of data, such as images or text, and then using that data to create new content. This process is often referred to as "deep learning," as the computer is able to learn from the data at a much deeper level than traditional machine learning algorithms.
Generative AI uses complex neural networks to analyze and learn patterns from the data it is fed. These neural networks are modeled on the structure and function of the human brain, with layers of interconnected nodes that process and analyze the input data. Through a process of trial and error, the neural network is able to refine its analysis and gradually learn to recognize and reproduce the patterns present in the data.
One of the key advantages of generative AI is its ability to create entirely new content that has not been seen before. This is achieved through a process known as "generative modeling," where the AI is trained to generate new content that is similar to the input data, but not identical. This can be used to create new images, videos, or even text that is similar in style and content to existing material, but with unique variations and combinations that make it distinct.
There are several types of generative AI models, each with their own strengths and weaknesses. For example, generative adversarial networks (GANs) use two neural networks, one to generate new content and another to evaluate and refine that content based on a set of predefined criteria. This can be used to create highly realistic images or videos, but may struggle with more complex data sets or abstract concepts.
Also Read: How Tata is becoming one of the Rated Data-Driven Company
Other types of generative AI models include variational autoencoders (VAEs), which use a probabilistic approach to generate new content, and recurrent neural networks (RNNs), which are designed for sequential data sets such as text or audio. Each of these models has its own unique features and applications, and the choice of model will depend on the specific use case and data set being analyzed.
Types of generative AI models
-
Generative Adversarial Networks (GANs)
-
Variational Autoencoders (VAEs)
-
Recurrent Neural Networks (RNNs)
-
Convolutional Neural Networks (CNNs)
-
Deep Belief Networks (DBNs)
-
Boltzmann Machines (BMs)
-
Auto-Regressive Models (ARMs)
-
Generative Flow Models
1. Generative Adversarial Networks (GANs):
GANs consist of two neural networks: a generator and a discriminator. The generator creates new data samples that are similar to the training data, while the discriminator tries to distinguish between the generated data and the real data. The generator improves over time as it tries to fool the discriminator, and this process continues until the generated data is indistinguishable from the real data.
2. Variational Autoencoders (VAEs):
VAEs are a type of neural network that learns to encode input data into a lower-dimensional space, and then generate new data samples from this encoded representation. VAEs use a probabilistic approach to generating new data, which allows for greater control over the characteristics of the generated data.
3. Recurrent Neural Networks (RNNs):
RNNs are a type of neural network that are used for sequential data, such as text or time-series data. RNNs are capable of learning the patterns and dependencies within the data, and can generate new data by sampling from the learned distribution.
4. Convolutional Neural Networks (CNNs):
CNNs are commonly used for image and video data. They use convolutional layers to extract features from the data, and then generate new data by sampling from the learned distribution.
5. Deep Belief Networks (DBNs):
DBNs are a type of neural network that consists of multiple layers of hidden units, with each layer learning increasingly complex representations of the data. DBNs are often used for unsupervised learning tasks, such as generating new data samples.
6. Boltzmann Machines (BMs):
BMs are a type of neural network that model the interactions between binary units in a probabilistic way. They can be used for a variety of tasks, including generating new data samples.
7. Auto-Regressive Models (ARMs):
ARMs are a type of generative model that generate new data samples by recursively predicting the next value in the sequence based on the previous values.
8. Generative Flow Models:
Generative flow models are a type of generative model that transform a simple distribution, such as a Gaussian distribution, into a more complex distribution that matches the characteristics of the training data. They can be used to generate new data samples by sampling from the learned distribution.
Also Read: Why AI is the Future of Marketing
Each of these types of generative AI models has its own strengths and weaknesses, and the choice of model will depend on the specific use case and data set being analyzed.
The Rise Of Generative AI And Its Effects On Video Platforms
Generative AI has been around for several years, but it has really taken off in recent years due to advances in computing power and the availability of large amounts of data. Many companies are now using generative AI to create new products and services, and the technology is being applied to a wide range of industries, including video platforms.
The effects of generative AI on video platforms are numerous and varied. Some of the most notable effects include:
1. Personalization
Generative AI can be used to create personalized content for users based on their preferences and viewing history. This can help video platforms to increase engagement and keep users coming back for more.
2. Content Creation
Generative AI can be used to create new video content automatically, reducing the time and resources required to produce high-quality videos. This can help video platforms to keep up with the demand for new content and stay ahead of the competition.
3. Enhanced Search and Discovery
Generative AI can be used to analyze video content and provide more accurate search and discovery results for users. This can help video platforms to improve the user experience and increase engagement.
4. Improved Advertising
Generative AI can be used to create more targeted and effective advertising campaigns. By analyzing user data, generative AI can create ads that are more likely to resonate with users and drive conversions.
5. Content Moderation
Generative AI can also be used to help with content moderation on video platforms. By analyzing video content and automatically flagging potentially inappropriate or harmful content, generative AI can help to reduce the risk of users being exposed to harmful content.
6. Cost Reduction
Generative AI can also help video platforms to reduce costs by automating tasks that would otherwise require human intervention. This can include tasks such as video editing, closed captioning, and translation.
7. Creative Assistance
Generative AI can assist creators in the video production process by providing suggestions and automating repetitive tasks such as adding transitions or creating captions. This can help to streamline the video production process and reduce the time and resources required to create high-quality content.
8. Copyright Protection
Generative AI can also be used to protect video platforms from copyright infringement. By analyzing video content and identifying potential copyright violations, generative AI can help video platforms to avoid legal issues and ensure that they are only hosting content that they have the rights to.
9. Improved Analytics
Generative AI can also provide video platforms with more detailed and accurate analytics by analyzing user behavior and providing insights into user preferences and engagement. This can help video platforms to make data-driven decisions and improve the user experience.
10. User-Generated Content Enhancement
Generative AI can be used to enhance user-generated content by adding effects, animations, or other enhancements automatically. This can help to make user-generated content more engaging and attractive to viewers.
11. Virtual Production
Generative AI can also be used in virtual production, where it can create realistic digital environments and characters. This can help to reduce the costs and time required for traditional film production, as well as provide new creative opportunities.
12. Deepfakes and Misinformation
Generative AI also presents challenges for video platforms in the form of deepfakes and misinformation. Deepfakes are synthetic videos that use generative AI to create realistic but fake content, while misinformation can be spread through the manipulation of video content. Video platforms must develop strategies to combat these issues while still leveraging the benefits of generative AI.
Overall, the effects of generative AI on video platforms are significant and far-reaching. While there are many potential benefits, there are also challenges that must be addressed in order to ensure that video platforms are able to provide high-quality content and protect their users.
Also Read: Morality and AI – The Real Struggle!
Potential Challenges and Risks
While generative AI has the potential to transform video platforms, there are also potential challenges and risks associated with the technology. Some of the most significant challenges include:
A. Quality Control
Generative AI can create content automatically, but there is always a risk that the quality of the content will be poor. This can lead to user dissatisfaction and damage the reputation of video platforms.
B. Ethics and Bias
Generative AI relies on large amounts of data, and there is a risk that the data used to train the algorithms may contain bias or perpetuate harmful stereotypes. This can lead to ethical concerns and damage the reputation of video platforms.
C. Regulatory Compliance
As with any new technology, there are potential regulatory compliance issues associated with the use of generative AI on video platforms. Video platforms will need to ensure that they are complying with relevant regulations and guidelines, such as data privacy laws.
Conclusion
Generative AI has the potential to revolutionize video platforms, but there are also potential challenges and risks associated with the technology. Video platforms will need to carefully consider these factors as they incorporate generative AI into their operations. However, with proper planning and implementation, generative AI could help video platforms to provide more personalized, engaging, and effective experiences for users.
You May Like
EDITOR’S CHOICE